In the age of data-driven decision-making, predictive betting algorithms have emerged as a game-changer in the world of sports and financial betting. These algorithms, powered by machine learning, statistics, and big data analytics, aim to tilt the odds in favor of the bettor—not by chance, but through calculated prediction lifecarehospitalthane.com. But how do these algorithms work, and what makes them effective?
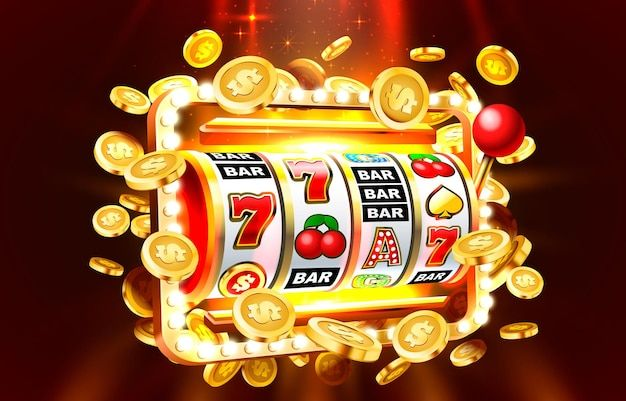
At their core, predictive betting algorithms are mathematical models designed to forecast the outcomes of events—typically sporting events, elections, or financial markets. Instead of relying on gut feeling or fandom, these algorithms analyze vast datasets to determine the probability of specific outcomes. This allows bettors to identify undervalued odds and place bets with positive expected value (EV).
The Key Components of a Predictive Betting Algorithm
- Data Collection
- Historical results (e.g., previous matches, player performance, weather conditions).
- Real-time updates (e.g., injuries, lineup changes, market movements).
- Behavioral and sentiment data (e.g., public opinion, betting volumes).
- Historical results (e.g., previous matches, player performance, weather conditions).
- Feature Engineering
- Translating raw data into meaningful inputs for the model. For example:
- A football team’s recent form.
- A player’s head-to-head statistics.
- The venue’s influence on game outcomes.
- A football team’s recent form.
- Translating raw data into meaningful inputs for the model. For example:
- Model Selection
- Common models include logistic regression, random forests, gradient boosting machines, and deep neural networks.
- More advanced setups might use ensemble methods or reinforcement learning to refine predictions over time.
- Common models include logistic regression, random forests, gradient boosting machines, and deep neural networks.
- Training and Testing
- The model is trained on historical data and validated on out-of-sample data to avoid overfitting.
- Cross-validation ensures robustness and generalization to future events.
- The model is trained on historical data and validated on out-of-sample data to avoid overfitting.
- Odds Evaluation
- The algorithm compares its predicted probabilities with bookmakers’ odds to identify discrepancies.
- Bets are placed only when there’s an edge—i.e., when the model’s implied probability is higher than the bookmaker’s.
- The algorithm compares its predicted probabilities with bookmakers’ odds to identify discrepancies.
Real-World Applications
- Sports Betting: From football and basketball to horse racing and esports, predictive algorithms are used to gain an edge in various markets.
- Financial Betting/Spread Betting: Traders use predictive models to forecast stock movements, commodity prices, and even cryptocurrency trends.
- Daily Fantasy Sports (DFS): Algorithms optimize lineups based on projections of player performance.
Limitations and Risks
While predictive betting algorithms can be powerful, they are not foolproof. Here are some important caveats:
- Market Efficiency: In highly liquid markets, bookmakers adjust odds quickly, reducing the opportunity for arbitrage.
- Data Quality: Poor or biased data leads to inaccurate predictions.
- Changing Dynamics: Sports and markets are dynamic—models must be regularly updated to remain relevant.
- Overfitting: A model too finely tuned to historical data may fail to generalize to new situations.
Ethical and Legal Considerations
As with any use of AI and automation, predictive betting brings up concerns:
- Fairness: Should bettors with advanced algorithms be allowed to compete against casual players?
- Addiction and Responsible Gambling: Predictive tools can exacerbate gambling problems if misused.
- Regulation: Jurisdictions may impose limits or licensing requirements for algorithmic betting systems.
The Future of Predictive Betting
With advancements in AI, particularly in real-time data processing and deep learning, predictive betting algorithms will become even more sophisticated. Integration with blockchain, live sensors, and even social media sentiment analysis could offer even more granular insights.
However, the human element—understanding context, recognizing anomalies, and making strategic decisions—still plays a vital role. The best results often come when human intuition and machine intelligence work together.
Predictive betting algorithms represent the intersection of mathematics, technology, and psychology. While they don’t guarantee winnings, they do offer a structured, analytical approach to a domain traditionally driven by luck and emotion. As long as they are used responsibly and ethically, these algorithms can be powerful tools in the hands of informed bettors.